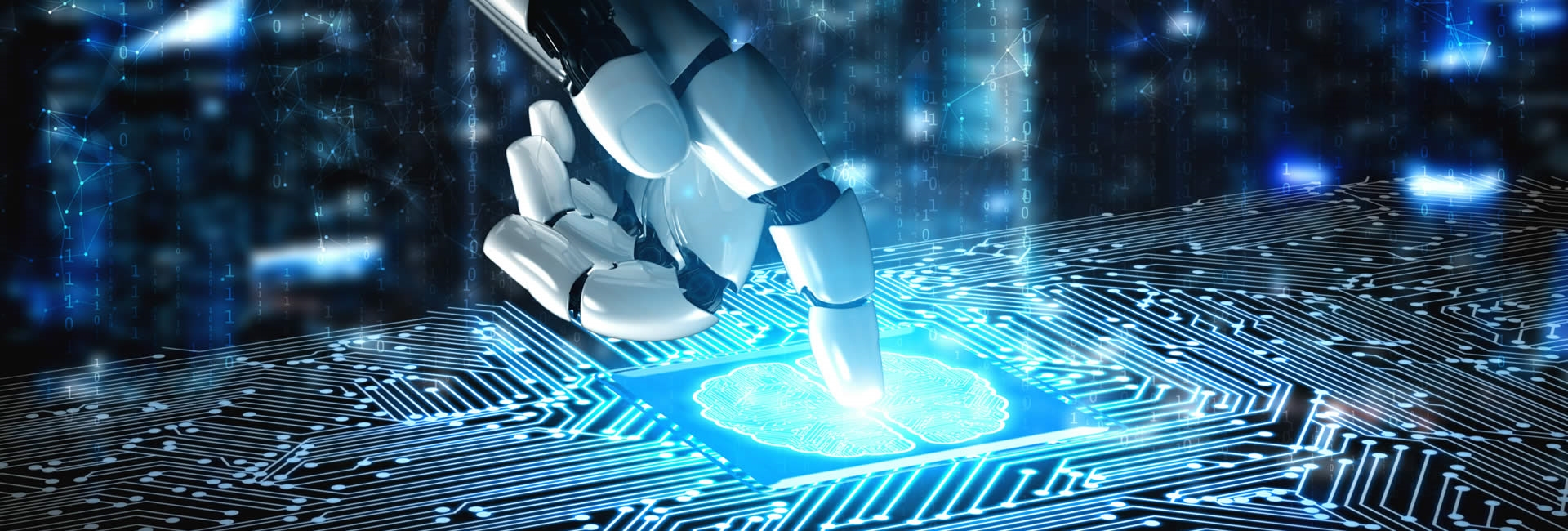
Topic
- Computing and Processing
- Components, Circuits, Devices and Systems
- Communication, Networking and Broadcast Technologies
- Power, Energy and Industry Applications
- Signal Processing and Analysis
- Robotics and Control Systems
- General Topics for Engineers
- Fields, Waves and Electromagnetics
- Engineered Materials, Dielectrics and Plasmas
- Bioengineering
- Transportation
- Photonics and Electrooptics
- Engineering Profession
- Aerospace
- Geoscience
- Nuclear Engineering
- Career Development
- Emerging Technologies
- Telecommunications
- English for Technical Professionals
Zichen Fan
Affiliation
University of Michigan, Ann Arbor, MI
Topic
Convolutional Neural Network,Energy Efficiency,Neural Network,Power Consumption,Convolutional Layers,Fully-connected Layer,Gated Recurrent Unit,Low Power Consumption,Neural Engineering,Sparse Weight,Datapath,Deep Neural Network,Dynamic Power,Energy Consumption,Fast Fourier Transform,Feature Maps,Internet Of Things,Keyword Spotting,Low-pass,Neural Network Classifier,Neural Network Processing,Non-volatile Memory,Non-zero Weights,Pulse Width,Reconfigurable Filter,Recurrent Neural Network,Reduction In Power,Settling Time,Sharp Transition,Sprinting,State Machine,System-on-chip,Top Left,Transconductance,Vision Tasks,28-nm CMOS,Adder Tree,Advances In Deep Learning,Analog-to-digital Converter,Application Programming Interface,Artificial Neural Network,Audio Interface,Autonomous Navigation,Bidirectional Recurrent Neural Network,Bit Error Rate,Boost Converter,Caching,Carrier Frequency,Carrier Phase,Change Detection,
Biography
Zichen Fan (Graduate Student Member, IEEE) received the B.S. degree from Tsinghua University, Beijing, China, in 2019. He is currently pursuing the Ph.D. degree with Michigan Integrated Circuit Laboratory, University of Michigan, Ann Arbor, MI, USA.
His current research interests include machine-learning accelerator design and efficient AI algorithm design, including model quantization, model pruning, and low-power VLSI digital system design.
His current research interests include machine-learning accelerator design and efficient AI algorithm design, including model quantization, model pruning, and low-power VLSI digital system design.