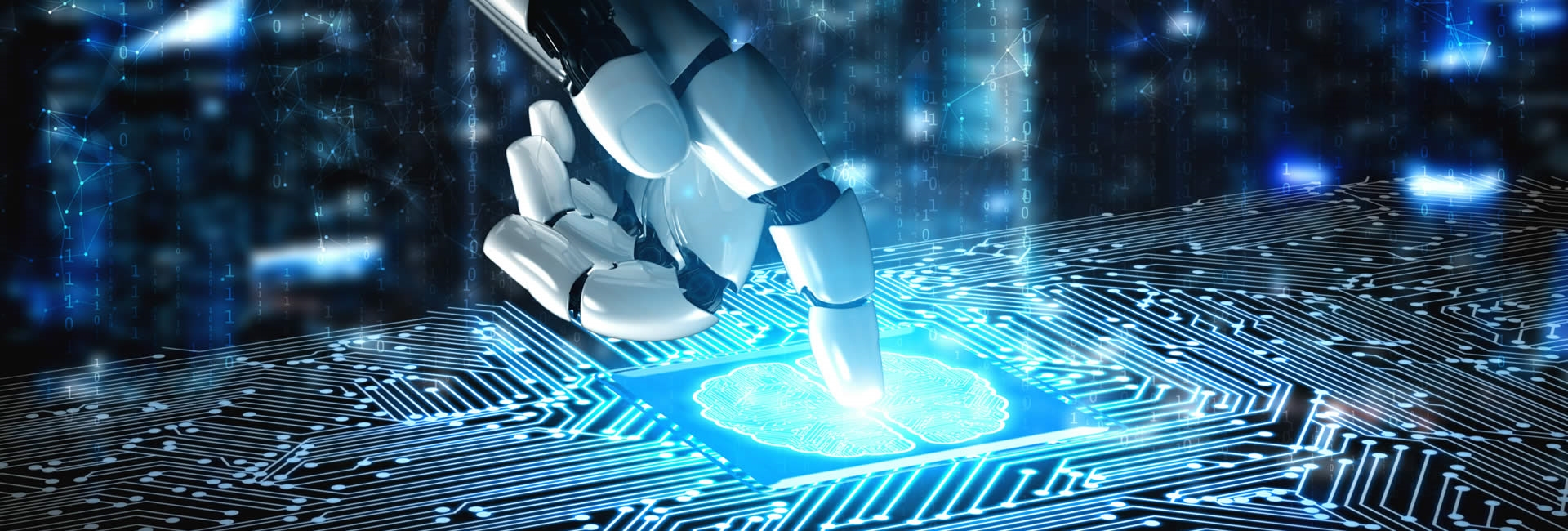
Topic
- Computing and Processing
- Components, Circuits, Devices and Systems
- Communication, Networking and Broadcast Technologies
- Power, Energy and Industry Applications
- Signal Processing and Analysis
- Robotics and Control Systems
- General Topics for Engineers
- Fields, Waves and Electromagnetics
- Engineered Materials, Dielectrics and Plasmas
- Bioengineering
- Transportation
- Photonics and Electrooptics
- Engineering Profession
- Aerospace
- Geoscience
- Nuclear Engineering
- Career Development
- Emerging Technologies
- Telecommunications
- English for Technical Professionals
Pierre Abillama
Affiliation
Department of Electrical Engineering and Computer Science, University of Michigan, Ann Arbor, MI, USA
Topic
Energy Efficiency,Neural Network,Convolutional Layers,Convolutional Neural Network,Energy Consumption,Fully-connected Layer,Neural Engineering,Artificial Neural Network,Datapath,Feature Maps,ImageNet,Neural Network Processing,Non-volatile Memory,Non-zero Weights,Sparse Weight,Accuracy-latency Trade-off,Adder Tree,Application Programming Interface,Audio Interface,Autonomous Navigation,Backpropagation Through Time,Balanced Trade-off,Caching,Challenging Dataset,Change Detection,Comparable Accuracy,Computation Energy,Computational Complexity,Convolution,Convolution Operation,Dataset Statistics,Deconvolution,Deep Neural Network,Dense Depth,Depthwise Convolution,Dynamic Datasets,Dynamic Power,Dynamic Power Consumption,Dynamic Vision Sensor,Element-wise Multiplication,Fast Fourier Transform,Feature Detection,Feature Tracking,Final Layer,Firing Threshold,Flow Data,Footprint,Fusion Layer,General-purpose Computer,Generative Adversarial Networks,
Biography
Pierre Abillama (Graduate Student Member, IEEE) received the B.Sc. degree in computer engineering from the University of Minnesota, Twin Cities, Minneapolis, MN, USA, in 2020, and the M.Sc. degree in electrical engineering from the University of Michigan, Ann Arbor, MI, USA, in 2022, where he is currently pursuing the Ph.D. degree in electrical engineering.
His current research interests include low-power and energy-efficient hardware accelerators and processors for deep learning and edge intelligence.
His current research interests include low-power and energy-efficient hardware accelerators and processors for deep learning and edge intelligence.