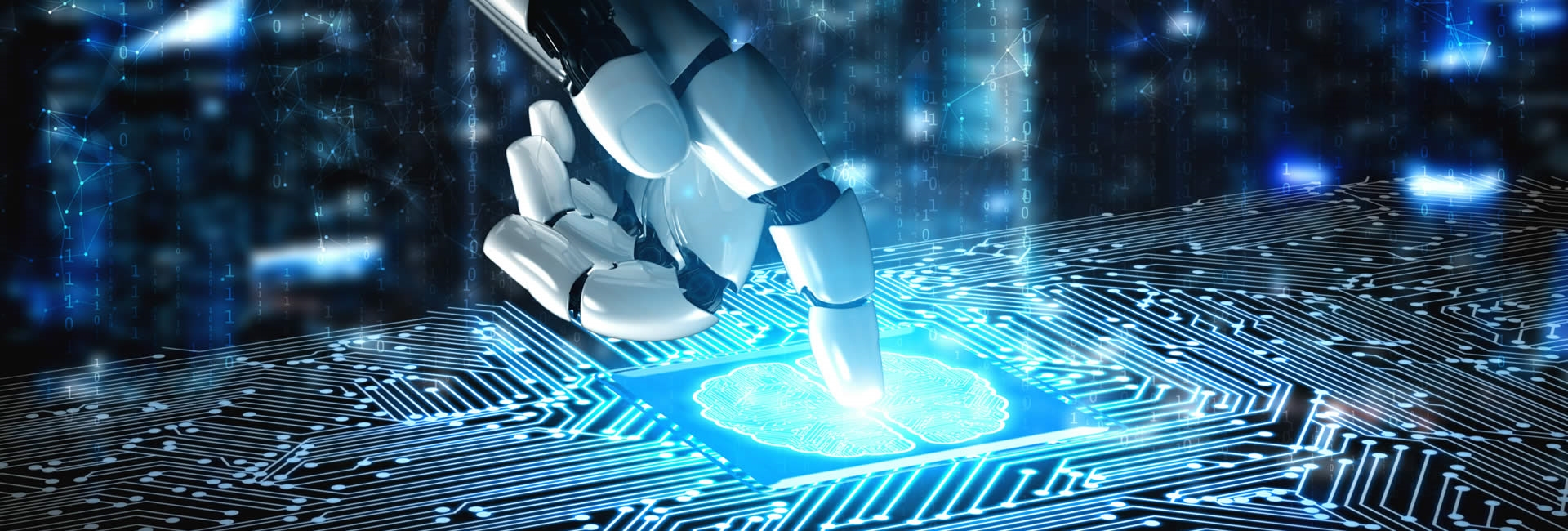
Topic
- Computing and Processing
- Components, Circuits, Devices and Systems
- Communication, Networking and Broadcast Technologies
- Power, Energy and Industry Applications
- Signal Processing and Analysis
- Robotics and Control Systems
- General Topics for Engineers
- Fields, Waves and Electromagnetics
- Engineered Materials, Dielectrics and Plasmas
- Bioengineering
- Transportation
- Photonics and Electrooptics
- Engineering Profession
- Aerospace
- Geoscience
- Nuclear Engineering
- Career Development
- Emerging Technologies
- Telecommunications
- English for Technical Professionals
Juan Castorena
Also published under:J. Castorena
Affiliation
Los Alamos National Lab, Los Alamos, U.S.A
Topic
Deep Learning,Neural Net,Convolutional Neural Network,Feature Learning,Mean Average Precision,Point Cloud,Batch Normalization,Binary Image,Fine-tuned,Image Retrieval,Learned Filters,MNIST Dataset,Natural Images,Node Features,Representation Learning,Retrieval Performance,Rigid Body Transformation,Stochastic Gradient Descent,Triplet Loss,3D Rotation,3D Translation,Adaptive Threshold Method,Additive Noise,Assumption Of Stationarity,Auxiliary Information,Best Practices,Challenging Problem,Chemical Composition,Classification Performance,Clean Data,Clear Signal,Complex Shapes,Computer Vision,Content-based Image Retrieval,Contrastive Loss,Convolution Theorem,Convolutional Layers,Data Reconstruction,Data Reduction,Deep Features,Deep Learning Approaches,Deep Learning Models,Deep Models,Delaunay Triangulation,Directed Acyclic Graph,Domain Generalization,Effect Of Noise,Effective Target,Element Oxides,Entire Image,
Biography
Juan Castorena (M'13) received the B.S. degree from the University of Texas at El Paso, in 2007 and M.S. degree in Biosystems Engineering from the University of Manitoba, Winnipeg, Canada in 2010. He is currently pursuing the Ph.D. degree in Electrical and Computer Engineering at New Mexico State University, Las Cruces.
His research interests include sub-Nyquist sampling, optimization, signal recovery, and LIDAR sampling and reconstruction.
His research interests include sub-Nyquist sampling, optimization, signal recovery, and LIDAR sampling and reconstruction.