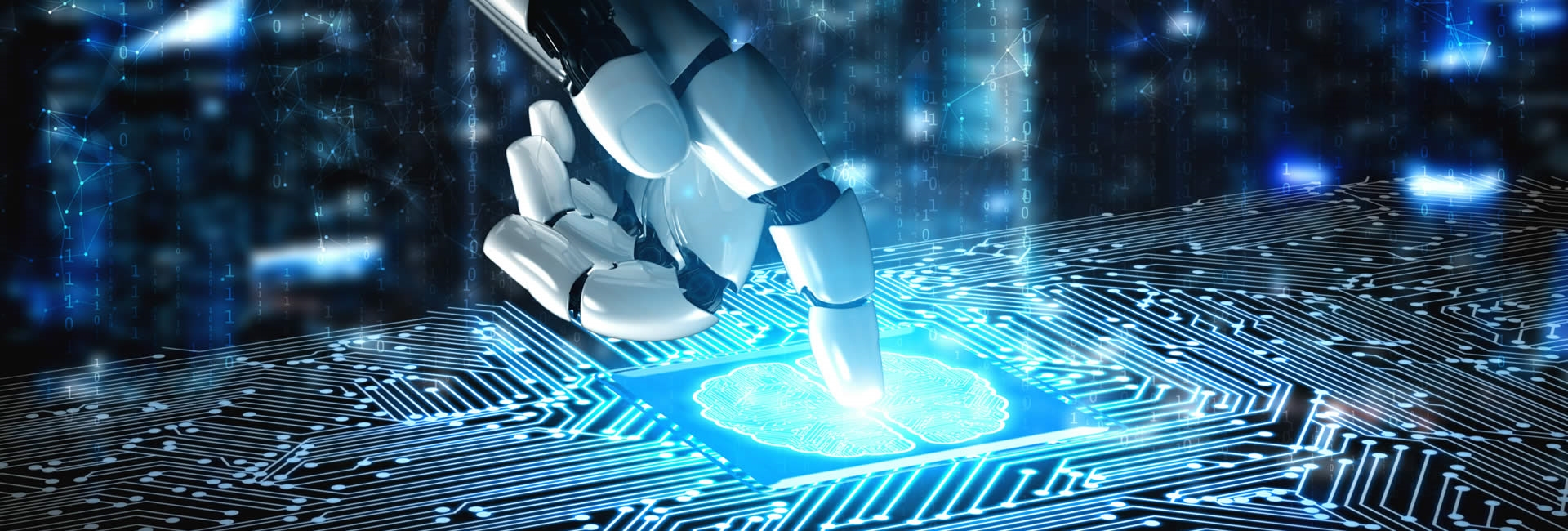
Topic
- Computing and Processing
- Components, Circuits, Devices and Systems
- Communication, Networking and Broadcast Technologies
- Power, Energy and Industry Applications
- Signal Processing and Analysis
- Robotics and Control Systems
- General Topics for Engineers
- Fields, Waves and Electromagnetics
- Engineered Materials, Dielectrics and Plasmas
- Bioengineering
- Transportation
- Photonics and Electrooptics
- Engineering Profession
- Aerospace
- Geoscience
- Nuclear Engineering
- Career Development
- Emerging Technologies
- Telecommunications
- English for Technical Professionals
Ammar Arshad
Also published under:
Affiliation
Faculty of Electrical Engineering, GIK Institute, Topi, Pakistan
Topic
Load Forecasting,Greenhouse Gas,Ensemble Model,Forecasting Model,Generation Expansion Planning,Load Consumption,Long Short-term Memory,Long Short-term Memory Model,Long Short-term Memory Network,Machine Learning,Machine Learning Models,Mean Absolute Error,Mean Absolute Percentage Error,Performance Metrics,Planning Horizon,Power Generation,Power Plants,Power System,Recurrent Neural Network,Renewable Energy Sources,Short-term Memory,Thermal Power Plants,1-minute Intervals,Accuracy Of Model,Actual Reward,Aggregate Data,Aggregate Demand,Average Demand,Base Case Scenario,Carbon Emission Reduction,Comparative Analysis,Computational Burden,Decision Node,Decision Tree,Decision Variables,Deep Neural Network,Deep Reinforcement Learning,Demand Response Programs,Distribution Network,Electric Power,Electricity Usage,Emission Reduction,Emission Spectra,Energy Consumption,Energy Demand,Energy Mix,Energy Source,Energy System,Energy Usage,Ensemble Technique,
Biography
Ammar Arshad (Member, IEEE) received the B.Sc. degree in electrical engineering from the University of Engineering and Technology, Lahore, Pakistan, in 2013, and the M.Sc. and D.Sc. degrees from Aalto University, Finland, in 2016 and 2019, respectively.
He has been with the Ghulam Ishaq Khan Institute of Engineering Sciences and Technology, since 2020. His main research interests include smart grid technologies’ utilization for PV integration in distribution networks, distributed voltage control, enhancement of PV hosting capacity, and demand response.
He has been with the Ghulam Ishaq Khan Institute of Engineering Sciences and Technology, since 2020. His main research interests include smart grid technologies’ utilization for PV integration in distribution networks, distributed voltage control, enhancement of PV hosting capacity, and demand response.